Intelligent Automation (IA) refers to different applications of Artificial Intelligence and other related technologies to streamline an organization’s processes, improving efficiency and identifying bottlenecks in the process. At the heart of IA’s evolutions lies process mining, a technique that provides insights into processes, identifies areas for improvement, and accelerates automation efforts. In this article, we will discuss how process mining is an important starting point for any IA project. This is the stepping stone to follow-up articles about the way AI can help automate processes intelligently.
IA encompasses a spectrum of modern AI technologies such as Machine Learning (ML) and robotic process automation (RPA). By leveraging these technologies, enterprises can automate repetitive tasks, gain valuable analytics, and empower decision-making capabilities efficiently. However, if you want to successfully automate your processes, you have to start with a good understanding of what they are and how they can be optimized, making process mining techniques a requirement for high-quality integration. Process mining can typically be broken down into 3 main phases:
- Discovery
- Monitoring
- Optimization
Discovery
Every organization has many operational business processes, often split between different domains. The discovery phase centers around uncovering, storing, and analyzing data from these processes. This often begins with the creation of event logs. An event log is a sequence of activities belonging to a particular process. These logs contain certain information about each step of a process. This information can vary in detail, but the minimum requirements are a case identification number (case ID), an activity description, and a timestamp. These event logs are collected and stored, typically in chronological order.
With a large enough sample of event logs, a process model can be created. Process models are the step-by-step outline of how a business operates from the inside. In other words, process models describe how a business process is carried out in its existing state. Lastly, these models are often visualized into easy-to-follow diagrams, giving everyone in the organization a clear overview of each process.
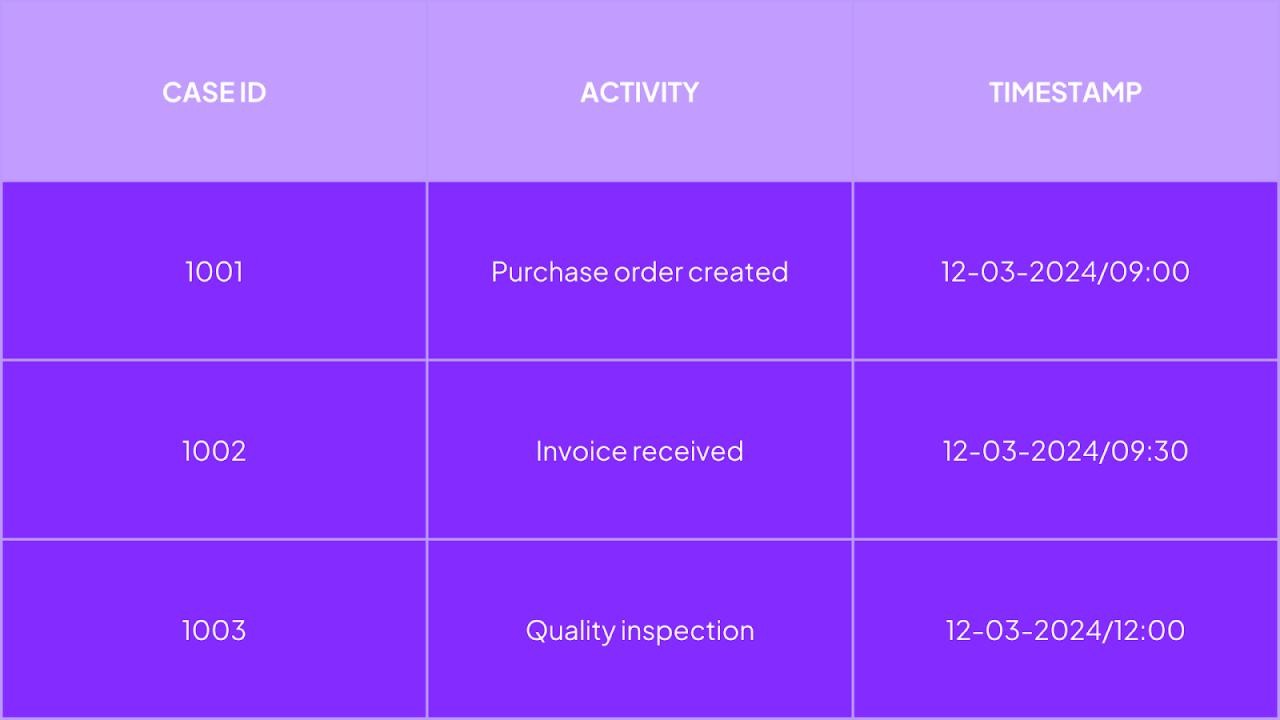
With a large enough sample of event logs, a process model can be created. Process models are the step-by-step outline of how a business operates from the inside. In other words, process models describe how a business process is carried out in its existing state. Lastly, these models are often visualized into easy-to-follow diagrams, giving everyone in the organization a clear overview of each process.
Monitoring
This phase consists mainly of comparing the process models with a reference model. A reference model is an optimized, ‘best case scenario’ version of a process model. These comparisons are called conformance checks. Performing a conformance check is a great way to uncover where hidden bottlenecks and breakdowns occur. You can use these findings to distinguish the root causes of inefficiencies throughout your business’ operations.
Through these steps an organization gathers, and sorts through, a lot of data. This data can be used alongside other technologies to create a Digital Twin of the organization. Digital Twins are virtual copies of a business. They mimic each process digitally. A Digital Twin offers the powerful ability to run simulations with changes to variables without any risk. In other words, a company can perform “trial and error” tests, without the risks associated with errors.
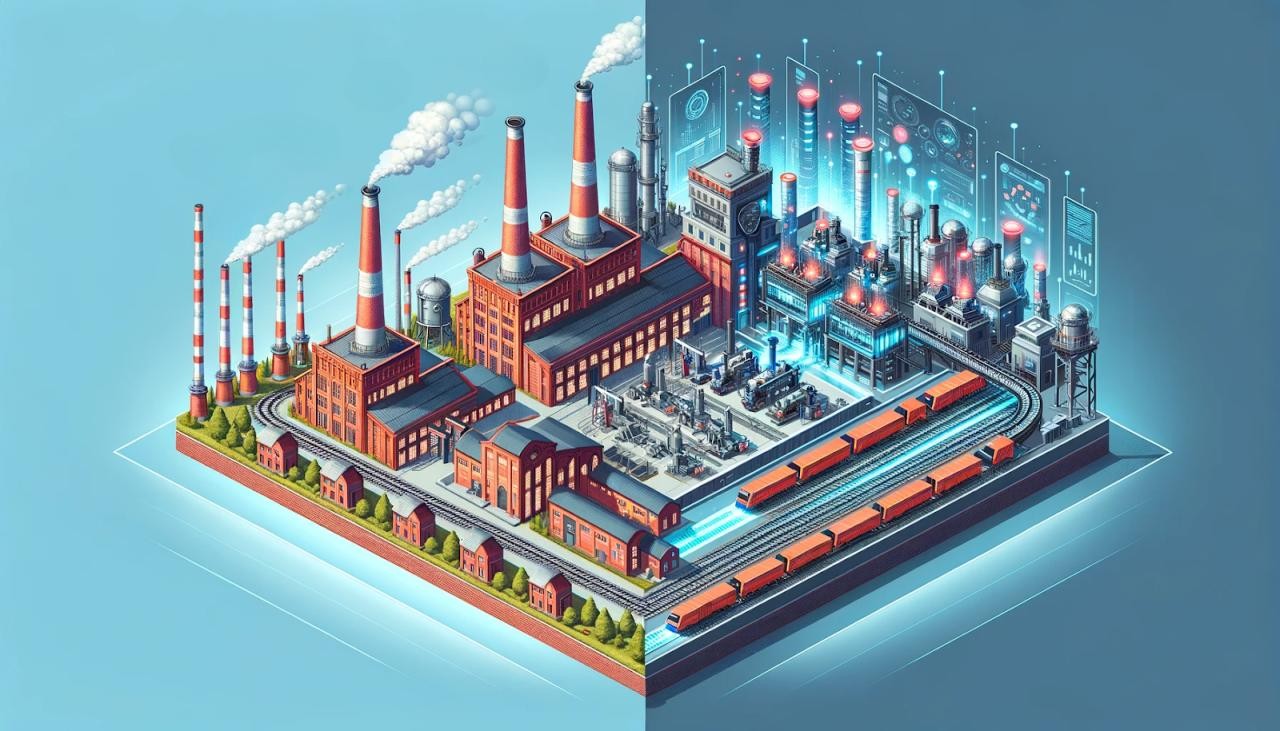
Optimization
Process mining’s third phase, optimization, mainly consists of such simulations. Here a business is free to run countless scenarios. Digital Twins offer complete freedom in simulating these outcomes, whether it would be more automation, having a different number of employees in a department, or any other change. This opens the possibility of prioritization based on statistics and data, as opposed to guesswork.
Iterate
Process mining is an iterative process where insights from the optimization and monitoring phases can lead back to further discovery and continuous improvement. Successful IA deployment requires careful planning, integration with existing systems, and ongoing management to ensure that the technologies effectively address the organization’s specific needs and challenges. Therefore, any organization looking to reap the benefits of these technologies needs to approach them cautiously. Consulting with professionals is crucial.
Into the future with IA
Intelligent Automation is developing at exponential speeds, thanks in no small part to process mining advancements. These technologies offer businesses unprecedented levels of efficiency. From discovering bottlenecks and points of friction throughout a business with conformance checks to endless simulations without tangible consequences through Digital Twins. Process mining within IA is a powerful tool that offers valuable insights that businesses can greatly benefit from. Although these advancements are exciting, adopters must be careful, as without proper planning and integration with existing systems the benefits of IA and process mining will be minimal.
Recent Comments